Assume the following simple regression model, Y = β0 + β1X + ϵ ϵ ∼ N(0, σ^2 ) Now run the following R-code to generate values of σ^2 = sig2, β1 = beta1 and β0 = beta0. Simulate the parameters using the following codes: Code: # Simulation ## set.seed("12345") beta0 <- rnorm(1, mean = 0, sd = 1) ## The true beta0 beta1 <- runif(n = 1, min = 1, max = 3) ## The true beta1 sig2 <- rchisq(n = 1, df = 25) ## The true value of the error variance sigmaˆ2 ## Multiple simulation will require loops ## nsample <- 10 ## Sample size n.sim <- 100 ## The number of simulations sigX <- 0.2 ## The variances of X # # Simulate the predictor variable ## X <- rnorm(nsample, mean = 0, sd = sqrt(sigX)) Q1 Fix the sample size nsample = 10 . Here, the values of X are fixed. You just need to generate ϵ and Y . Execute 100 simulations (i.e., n.sim = 100). For each simulation, estimate the regression coefficients (β0, β1) and the error variance (σ 2 ). Calculate the mean of the estimates from the different simulations. What did you expect the mean to be? Plot the histogram of each of the regression parameter estimates from (b). Explain the pattern of the distributions. Obtain the variance of the regression parameter estimator (i.e., βˆ 0 and βˆ 1) from the simulations. That is, calculate the sample variances of the regression parameter estimates from the 100 simulations. Is this variance approximately equal to the true variances of the regression parameter estimates? Construct the 95% t and z confidence intervals for β0 and β1 during every simulation. What is the proportion of the intervals for each method containing the true value of the parameters? Is this consistent with the definition of confidence interval? Next, what differences do you observe in the t and z confidence intervals? What effect does increasing the number of simulations from 100 have on the confidence intervals? For steps (a)-(d) the sample size was fixed at 10. Start increasing the sample size (e.g., 20, 50, 100) and run steps (a)-(d). Explain what happens to the mean, variance and distribution of the estimators as the sample size increases. Choose the largest sample size you have used in step (f). Fix the sample size to that and start changing the error variance (sig2). You can increase and decrease the value of the error variance. For each value of error variance execute steps (a) - (d). Explain what happens to the mean, variance and distribution of the estimates as the error variance changes.
Assume the following simple regression model, Y = β0 + β1X + ϵ ϵ ∼ N(0, σ^2 ) Now run the following R-code to generate values of σ^2 = sig2, β1 = beta1 and β0 = beta0. Simulate the parameters using the following codes: Code: # Simulation ## set.seed("12345") beta0 <- rnorm(1, mean = 0, sd = 1) ## The true beta0 beta1 <- runif(n = 1, min = 1, max = 3) ## The true beta1 sig2 <- rchisq(n = 1, df = 25) ## The true value of the error variance sigmaˆ2 ## Multiple simulation will require loops ## nsample <- 10 ## Sample size n.sim <- 100 ## The number of simulations sigX <- 0.2 ## The variances of X # # Simulate the predictor variable ## X <- rnorm(nsample, mean = 0, sd = sqrt(sigX)) Q1 Fix the sample size nsample = 10 . Here, the values of X are fixed. You just need to generate ϵ and Y . Execute 100 simulations (i.e., n.sim = 100). For each simulation, estimate the regression coefficients (β0, β1) and the error variance (σ 2 ). Calculate the mean of the estimates from the different simulations. What did you expect the mean to be? Plot the histogram of each of the regression parameter estimates from (b). Explain the pattern of the distributions. Obtain the variance of the regression parameter estimator (i.e., βˆ 0 and βˆ 1) from the simulations. That is, calculate the sample variances of the regression parameter estimates from the 100 simulations. Is this variance approximately equal to the true variances of the regression parameter estimates? Construct the 95% t and z confidence intervals for β0 and β1 during every simulation. What is the proportion of the intervals for each method containing the true value of the parameters? Is this consistent with the definition of confidence interval? Next, what differences do you observe in the t and z confidence intervals? What effect does increasing the number of simulations from 100 have on the confidence intervals? For steps (a)-(d) the sample size was fixed at 10. Start increasing the sample size (e.g., 20, 50, 100) and run steps (a)-(d). Explain what happens to the mean, variance and distribution of the estimators as the sample size increases. Choose the largest sample size you have used in step (f). Fix the sample size to that and start changing the error variance (sig2). You can increase and decrease the value of the error variance. For each value of error variance execute steps (a) - (d). Explain what happens to the mean, variance and distribution of the estimates as the error variance changes.
Operations Research : Applications and Algorithms
4th Edition
ISBN:9780534380588
Author:Wayne L. Winston
Publisher:Wayne L. Winston
Chapter12: Review Of Calculus And Probability
Section12.5: Random Variables, Mean, Variance, And Covariance
Problem 5P
Related questions
Question
Assume the following simple regression model,
Y = β0 + β1X + ϵ
ϵ ∼ N(0, σ^2 )
Now run the following R-code to generate values of σ^2 = sig2, β1 = beta1 and β0 = beta0. Simulate the parameters using the following codes:
Code:
# Simulation ##
set.seed("12345")
beta0 <- rnorm(1, mean = 0, sd = 1) ## The true beta0
beta1 <- runif(n = 1, min = 1, max = 3) ## The true beta1
sig2 <- rchisq(n = 1, df = 25) ## The true value of the error variance sigmaˆ2
## Multiple simulation will require loops ##
nsample <- 10 ## Sample size n.sim <- 100 ## The number of simulations
sigX <- 0.2 ## The variances of X #
# Simulate the predictor variable ##
X <- rnorm(nsample, mean = 0, sd = sqrt(sigX))
Q1
- Fix the sample size nsample = 10 . Here, the values of X are fixed. You just need to generate ϵ and Y . Execute 100 simulations (i.e., n.sim = 100). For each simulation, estimate the regression coefficients (β0, β1) and the error variance (σ 2 ). Calculate the mean of the estimates from the different simulations. What did you expect the mean to be?
- Plot the histogram of each of the regression parameter estimates from (b). Explain the pattern of the distributions.
- Obtain the variance of the regression parameter estimator (i.e., βˆ 0 and βˆ 1) from the simulations. That is, calculate the sample variances of the regression parameter estimates from the 100 simulations. Is this variance approximately equal to the true variances of the regression parameter estimates?
- Construct the 95% t and z confidence intervals for β0 and β1 during every simulation. What is the proportion of the intervals for each method containing the true value of the parameters? Is this consistent with the definition of confidence interval? Next, what differences do you observe in the t and z confidence intervals? What effect does increasing the number of simulations from 100 have on the confidence intervals?
- For steps (a)-(d) the sample size was fixed at 10. Start increasing the sample size (e.g., 20, 50, 100) and run steps (a)-(d). Explain what happens to the mean, variance and distribution of the estimators as the sample size increases.
- Choose the largest sample size you have used in step (f). Fix the sample size to that and start changing the error variance (sig2). You can increase and decrease the value of the error variance. For each value of error variance execute steps (a) - (d). Explain what happens to the mean, variance and distribution of the estimates as the error variance changes.
Expert Solution

This question has been solved!
Explore an expertly crafted, step-by-step solution for a thorough understanding of key concepts.
Step by step
Solved in 2 steps

Knowledge Booster
Learn more about
Need a deep-dive on the concept behind this application? Look no further. Learn more about this topic, computer-science and related others by exploring similar questions and additional content below.Recommended textbooks for you
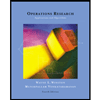
Operations Research : Applications and Algorithms
Computer Science
ISBN:
9780534380588
Author:
Wayne L. Winston
Publisher:
Brooks Cole
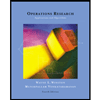
Operations Research : Applications and Algorithms
Computer Science
ISBN:
9780534380588
Author:
Wayne L. Winston
Publisher:
Brooks Cole